Introduction
Active pharmaceutical ingredient (API) manufacturing is a critical foundation of the pharmaceutical industry, responsible for creating the potent compounds that drive the effectiveness of countless medications. This process is highly specialized, involving complex chemical syntheses, precise reaction conditions, and rigorous purification steps to ensure that each API meets stringent quality and potency standards.
In recent years, however, the landscape of small molecule manufacturing has grown increasingly intricate. Producers face mounting challenges, not only from regulatory and market pressures but also due to the complexity of the chemical processes involved in synthesizing these APIs.1
As these challenges intensify, the need for streamlined and optimized production becomes crucial. This is where artificial intelligence (AI) enters the equation, transforming small molecule manufacturing by improving operational efficiency, enhancing quality control, and ultimately delivering more consistent and effective medications for patients.
Overview of Small Molecule Production Process
The small molecule production process is multifaceted, involving several critical stages, each requiring specialized techniques and equipment. In the following sections, we’ll take a look at ibuprofen to exemplify a small molecule production process.
The History of Ibuprofen
Ibuprofen, a widely used nonsteroidal anti-inflammatory drug (NSAID) and marketed under names such as Advil® and Motrin®., was first patented in 1961 by Boots Pure Chemical Company and became available over-the-counter in 1984. At this time, the synthesis or development process, processes took 6 steps.2

The Hoechst process for ibuprofen synthesis, developed by the Boots-Hoechst-Celanese (BHC) company, was a significant improvement over the original Boots process, reducing the synthesis to three steps:
- Friedel-Crafts acylation of isobutylbenzene using HF as catalyst
- Catalytic hydrogenation to reduce the ketone to an alcohol
- Carbonylation with carbon monoxide using a palladium catalyst
This process is more environmentally friendly, producing only water as a by-product.3
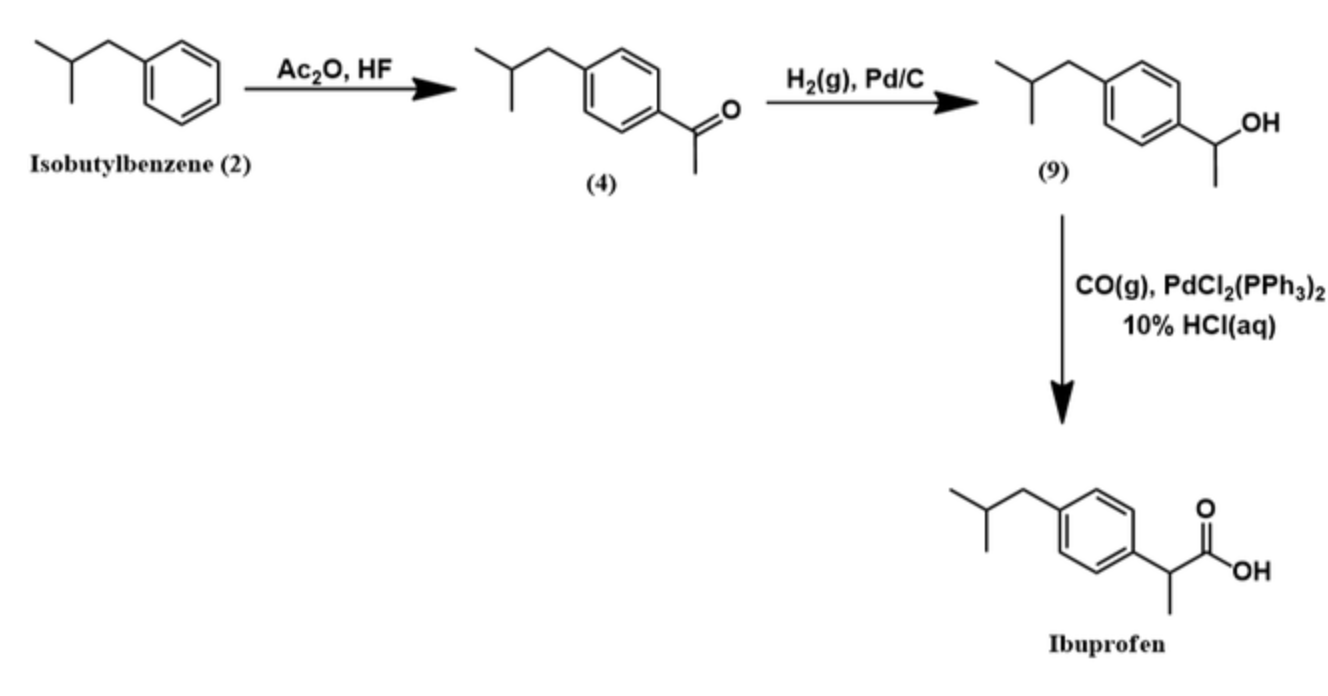
Today, manufacturers of ibuprofen continue to improve the development processes for the drug. The constant process improvement within API manufacturing is not just exclusive to ibuprofen but encompasses many existing and new drug therapies.
With AI, this process improvement can be achieved at each stage of the drug development process, creating a faster, more efficient delivery of essential medications. Let’s explore each stage in depth.
Small Molecule Lab Scale Overview
The first step in small molecule manufacturing is lab-scale process characterization and development, which serves as the initial exploratory phase. At this stage in the manufacturing of ibuprofen, researchers would have determined the feasibility of producing the small molecule.
This lab work allows chemists to experiment with different reaction conditions to synthesize a new drug. For ibuprofen, this might include studying the chemical reaction where isobutylbenzene is converted into ibuprofen.4
In small molecule production, Design of Experiments (DoE) are used to understand how different materials and process variables affect the quality of a drug. Researchers can systematically test variables such as temperature and reagent quantities to identify optimal conditions. Through statistical analysis, DoE helps to assess the impact of each variable on the desired outcomes, identifying critical factors essential for successful production. Once these critical factors are pinpointed, DoE optimizes their levels to establish ideal conditions, laying a strong foundation for scaling up the production process efficiently.5
Traditional lab-scale development is often hindered by challenges such as limited resources, long cycle times, significant experimental burden, and the inability to foresee issues that may arise during scaling.6
AI-driven API Process Development at the Lab Scale
Modeling and simulations play a crucial role in supporting the manufacturing of small molecule active pharmaceutical ingredients (APIs) at the lab scale by enhancing the efficiency, accuracy, and predictability of process development.
One significant contribution of modeling is in virtual experimentation and optimization. Researchers can virtually simulate various reaction conditions, such as temperature, pressure, and reagent concentrations, to identify the optimal conditions for small molecule manufacturing. This approach minimizes the number of experiments required, which accelerates the overall development timeline.7, 8
Small Molecule Pilot Production Overview
The next phase is pilot-scale production, which acts as a crucial transition from lab-scale experimentation to full-scale manufacturing. This stage tests the viability of processes on a larger scale and serves to validate the methodologies developed during the lab phase.9
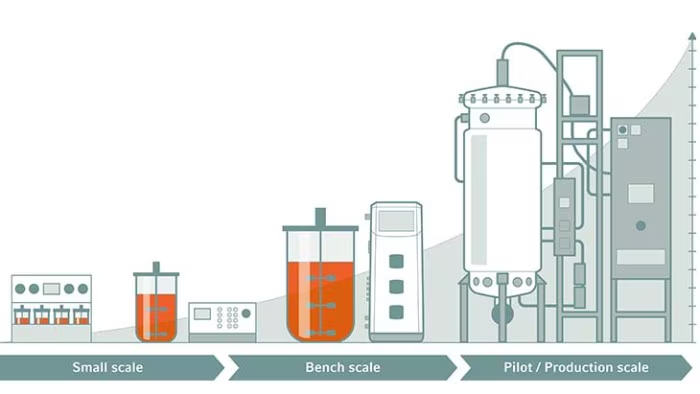
In ibuprofen’s case, pilot-scale production involves scaling up the chemical reaction that creates ibuprofen and testing how well it performs under larger batch conditions, such as 500 g/day. The transition from lab scale to pilot scale is crucial, as new factors like heat transfer and mixing dynamics can affect the reaction outcomes.10
Pilot-scale production is not merely an extension of lab work; it is a necessary step to ensure that the processes can be successfully scaled up without significant deviations in quality or yield. During this phase, manufacturers face specific challenges related to the consistency of reaction yields and the maintenance of product quality. These challenges necessitate a thorough understanding of both the chemical processes involved and the equipment used.11
AI-Driven Predictive Scale up at Small Molecule Pilot Scale
Model-driven simulations enable engineers to simulate the scale-up process, thereby reducing the number of trial runs and resources consumed in the scale-up process. Model predictive scale-up allows researchers to anticipate potential challenges, such as yield variability or impurities, before they arise in physical experiments. By addressing these issues early on, manufacturers can streamline their processes and improve the efficiency of small molecule API production.8

An Overview of Small Molecule Commercial Scale Production
Following the pilot stage is commercial-scale production, where achieving process consistency is paramount. This is where a drug like ibuprofen is produced on a massive scale for global distribution, with annual production estimated at approximately 45,000 tons. The objective here is to maintain consistency across every batch of the API, ensuring that each ibuprofen pill or tablet performs as expected.12
In large-scale production, even slight deviations in reaction conditions or material quality can lead to significant issues, from inefficiencies to quality deviations. These issues can have far-reaching repercussions, from regulatory penalties and increased production costs to potential harm to a company's reputation.13
Therefore, the need for effective quality control mechanisms is critical. Challenges at this stage include managing complex supply chains, ensuring thorough quality assessments, and controlling costs to maintain profitability.
AI-driven Continuous Process Verification at the Commercial Scale
The role of AI becomes even more pronounced at the commercial scale, where it can enable continuous process verification, and optimize control setpoints mid-batch ultimately enhancing operational efficiency. By leveraging sensor data, AI-powered systems continuously monitor production processes, enabling early fault detection and minimizing disruptions. These advanced monitoring and control systems ensure that manufacturing remains within defined parameters, allowing for real-time adjustments that uphold product quality. 14, 15
Predictive modeling further optimizes manufacturing processes by comparing real-time data with historical data from previous batches, this is often called Process Fingerprinting. This capability enables the detection of outliers, ultimately leading to significant improvements in product quality and performance.7
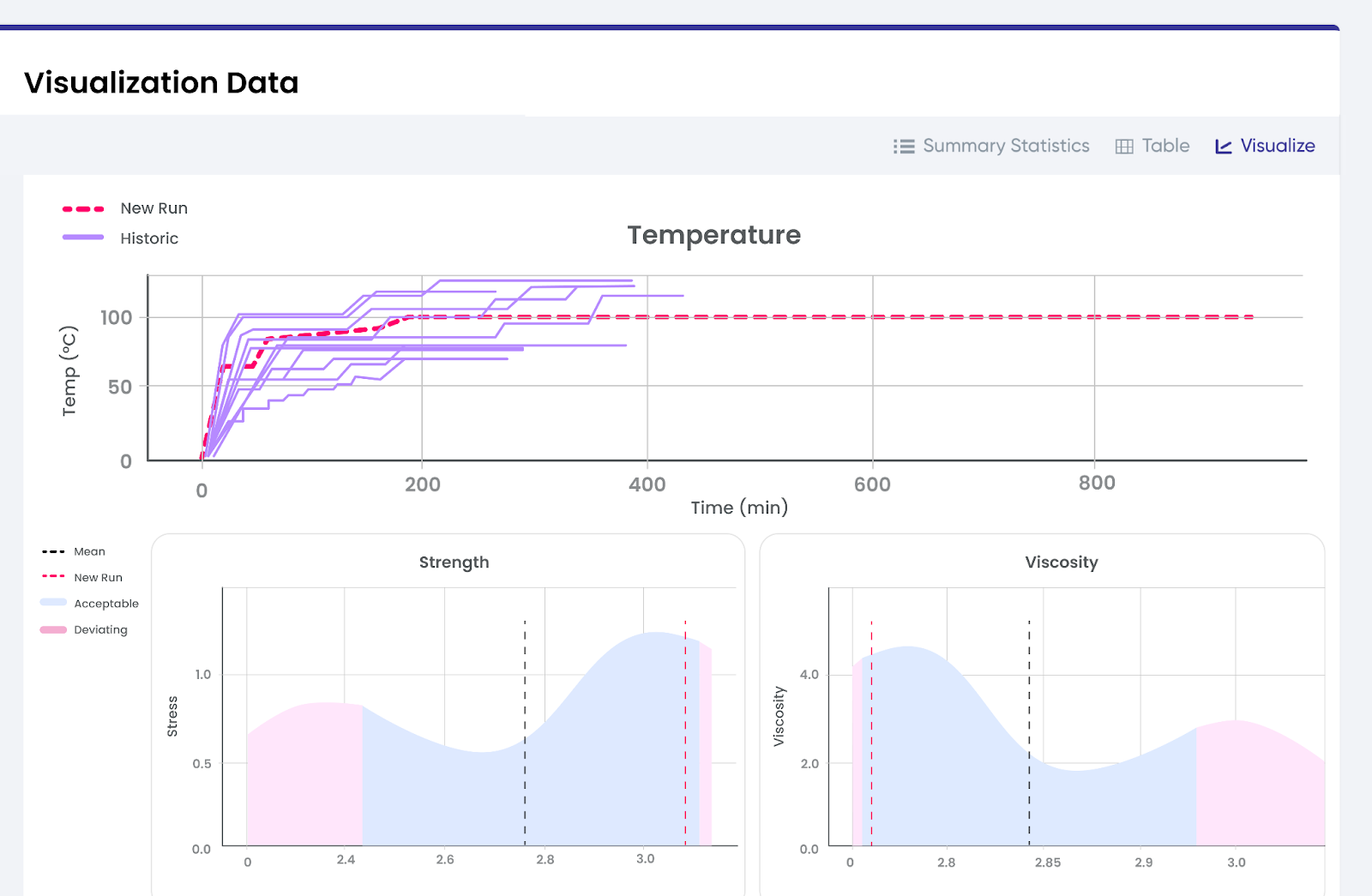
This technology not only enhances efficiency and output but also helps to safeguard product integrity, which is critical in the highly regulated pharmaceutical landscape.
Future Trends and Innovations: API Manufacturing
Looking ahead, the potential for AI to further revolutionize small molecule, API manufacturing is vast. Emerging technologies, such as "lights out" facilities, which operate autonomously with minimal human intervention, are expected to enhance both production efficiency and quality control.
Another innovative application of AI in manufacturing is the use of Generative AI for advanced search and retrieval. For example, a pharmaceutical company could use Gen AI to analyze historical reaction yield data, optimizing critical parameters such as temperature, pH, and solvent selection for small molecule synthesis. During scale-up, it could retrieve insights about equipment constraints and process safety considerations from past projects, helping reduce cycle time and ensuring process reliability.16
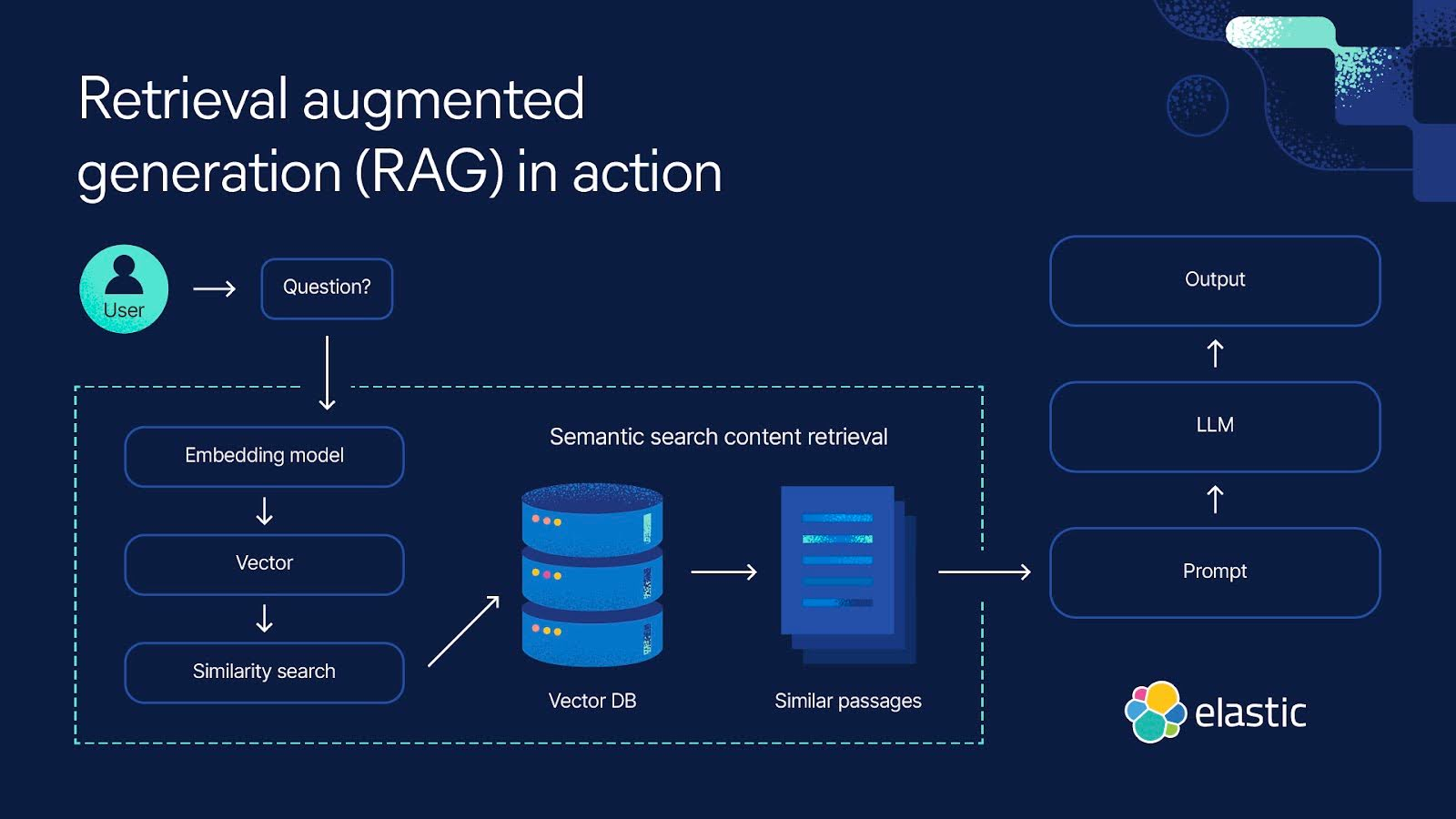
As AI continues to advance, predictive analytics and real-time monitoring will likely become standard practices within the industry, allowing manufacturers to respond swiftly to potential issues and maintain operational excellence.
Conclusion
The integration of AI into small molecule API manufacturing represents a significant shift in the industry's approach to production processes. By leveraging AI's capabilities, manufacturers can navigate the increasing complexities of small molecule production more effectively, achieving higher efficiency, improved consistency, and ultimately, better patient outcomes.
As the technology continues to evolve, the future of small molecule manufacturing looks promising, with AI at the forefront, guiding the industry toward a more streamlined and efficient production paradigm.
Ready to see how Basetwo's AI platform can transform your small molecule manufacturing process? Book a demo today and explore the possibilities.