Industrial manufacturers are constantly seeking ways to enhance efficiency, reduce costs, and boost productivity all while staying ahead of their competitors. An opportunity to bolster these efforts is AI-enabled optimization—a concept that goes beyond traditional process improvement methods like Lean Six Sigma to incorporate AI models. By leveraging model-based optimization, manufacturers can significantly impact their profitability and productivity.

What is AI-Driven Optimization?
Optimization in industrialmanufacturing is all about improving processes to achieve better outcomes. Traditionally, this has involved methods such as Lean Six Sigma, which focus on reducing waste and improving quality. However, AI-driven optimization takes this a step further by using predictive models and simulations to make data-driven decisions that enhance efficiency, quality, and profitability.
Model-based optimization combines traditional process improvement approaches with predictive algorithms. This allows manufacturers to simulate various scenarios and determine the optimal decisions for running processes more efficiently. The integration of AI into these models enables a more holistic approach to optimization, ensuring that all aspects of the manufacturing process are considered and improved upon.
Reinforcement Learning in Optimization
One powerful AI technique used in optimization is reinforcement learning. This method uses an iterative approach to converge on optimal decisions by rewarding good actions and penalizing bad ones. For example, in the context of a self-driving car, the car learns to make optimal driving decisions by receiving feedback on its actions – rewarding it when successfully changing lanes, and penalizing it when not stopping at a stop sign. This same principle can be applied to manufacturing processes, such as optimizing the operation of a heat exchanger to save energy or a mixer to improve fluid distribution.
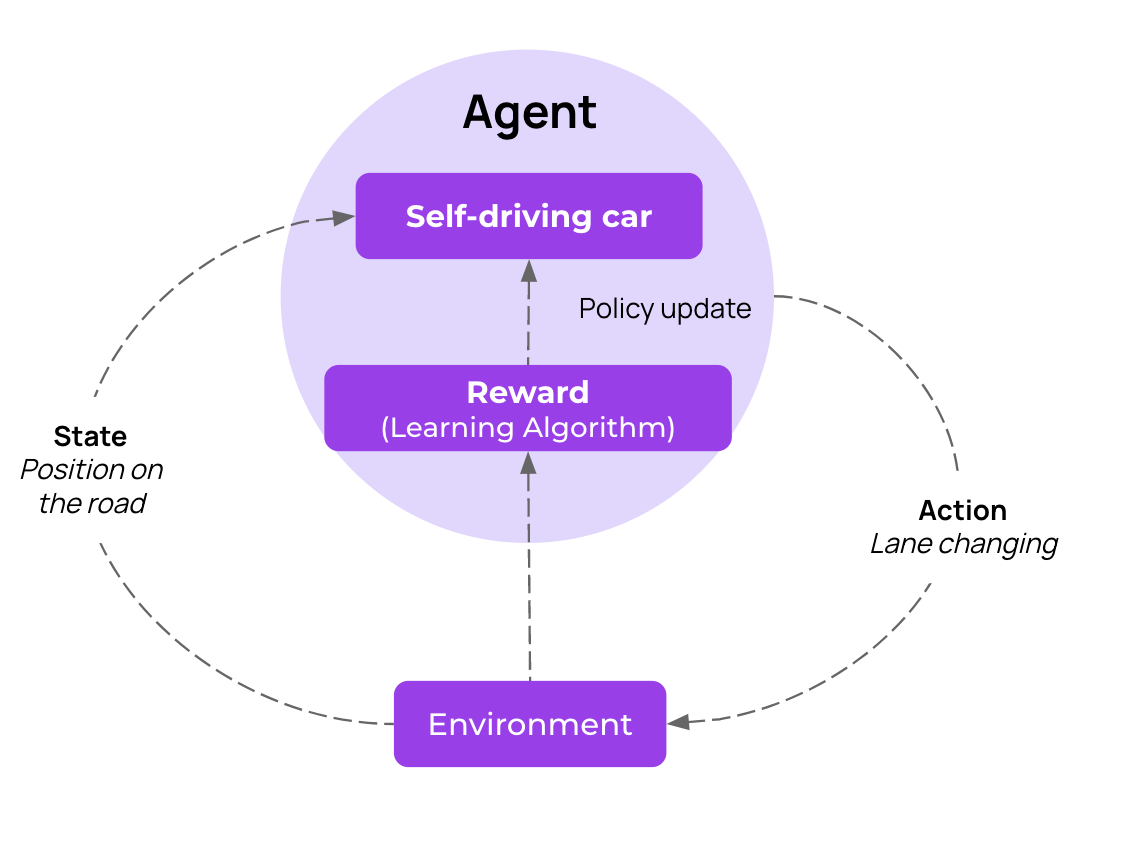
Applications of AI in Process Engineering and Manufacturing
Fault Detection and Maintenance Optimization
In many manufacturing environments, fault detection systems alert engineers when something goes wrong, but they often fail to provide solutions. AI-driven optimization can layer on top of these systems to offer actionable recommendations. For instance, instead of merely indicating a problem with a filtration membrane or heat exchanger, the system can suggest scheduling maintenance during downtime to fix the issue efficiently.

There are two main scopes of maintenance optimization solutions:
- Fault Detection and Root Cause Analysis: Provides insight to engineers about potential equipment issues, their respective failure modes, and steps they can take to resolve identified issues at the unit operational level.
- Optimized Maintenance Plans: Offers holistic, plant-wide maintenance schedules, to reduce unplanned asset downtime and the total cost of ownership of mission-critical assets.
The results of implementing such optimization solutions can be significant, including reductions in downtime by up to 50%, increases in throughput by 30%, and decreases in costs associated with producing high-quality products by up to 20%.
Process Control Optimization
AI-driven optimization is also transforming how processes are controlled across the manufacturing industry. Manufacturers face challenges such as long cycle times, high raw material consumption, and energy usage. By using digital twins—virtual replicas of physical processes—manufacturers can simulate changes and optimize inputs to achieve better outcomes.
For example, a digital twin of a reactor can simulate different feed rates and control set points to predict key performance indicators (KPIs) related to yield and quality in distillation columns or mixers. This allows for optimization strategies ranging from minor adjustments to creating entirely new standard operating procedures (SOPs). The impact can include reductions in cycle time, material usage, and production costs by up to 40%, and energy consumption by up to 20%.

Energy Efficiency
AI-driven optimization can also improve energy efficiency in manufacturing, oil refineries or drilling rigs, where fossil-fueled generators or cooling water systems are often run conservatively due to a lack of real-time data. By creating a model of the site’s power demand and its activities, and applying reinforcement learning algorithms, operators can optimize generator or chiller loads to reduce fuel/energy consumption and emissions. In this example, the recommendation produced by the AI algorithm was to operate fewer fossil-fueled generators at a higher load set point. Companies have seen annual savings of over $500,000 in fuel costs per rig and reductions of over 1,500 tons of CO2 emissions per site with AI-enabled optimization.

The Future of AI-Enabled Optimization
The future of optimization lies in making these advanced techniques more accessible and impactful. Generative AI, for instance, can simplify the implementation of model-based optimization by allowing engineers to write objective functions without any coding experience. This democratizes the use of advanced optimization methods, making them accessible to a broader range of engineers.
Moreover, the development of autonomous plants (“lights out facilities”), where AI systems can autonomously implement control commands in real-time without human intervention, represents a significant leap forward. These systems can handle routine optimizations autonomously, allowing engineers to manage several facilities from a centralized, remote monitoring site.
Conclusion
AI-driven optimization offers a powerful toolset for manufacturers looking to enhance efficiency, reduce costs, and stay competitive. By leveraging data-driven solutions and actionable recommendations, manufacturers can achieve significant improvements in productivity and profitability. At Basetwo, we are dedicated to helping engineers navigate these complexities with our AI copilot, supporting everything from data analysis to model-based optimization.
For more insights on how to go about optimizing your processes, explore our platform or book a demo with us directly for a more tailored experience.