Introduction
Oral solid dose (OSD) manufacturing remains one of the most widely used and commercially important forms of drug manufacturing in the pharmaceutical industry. From over-the-counter pain relievers to complex cancer treatments, tablets and capsules represent a cornerstone of modern medicine. Despite their apparent simplicity, producing oral solid doses at scale requires incredible precision to ensure product consistency, quality, and regulatory compliance.
Yet, pharmaceutical manufacturers continue to face significant challenges in achieving consistent output. Variability introduced during processes like granulation or blending can compromise the efficacy, bioavailability, and shelf life of the final product. These inconsistencies not only affect therapeutic outcomes but can also trigger costly recalls or production halts under strict GMP regulations.1
Artificial intelligence (AI) and digital twins are emerging as key enablers for pharmaceutical manufacturers seeking to reduce process variability, improve quality control, and maintain consistent quality outcomes. By moving beyond traditional data monitoring to real-time predictions and actionable recommendations, AI is ushering in a new era of safe, consistent, and reliable OSD manufacturing.
The Root Causes of Inconsistency in OSD Manufacturing
Achieving uniformity in OSD manufacturing is complex due to the sheer number of variables that influence product quality. These inconsistencies often originate upstream, during the raw material and early process stages such as synthesis, where they can cascade into downstream issues if not proactively addressed.
Raw material variability is a leading contributor. Even when working with the same active pharmaceutical ingredient (API) and excipients, slight differences in particle size distribution, moisture content, flowability, or bulk density can have a significant impact on blend uniformity and compression behavior.2 These physical attributes can change based on supplier, lot, or storage conditions, making it difficult to predict their impact using conventional tools.
Process deviations are another critical source of inconsistency. OSD manufacturing involves a series of tightly linked operations—including granulation, blending, tableting, and coating—each of which can introduce variability in the final product quality.

Granulation, for example, is influenced by factors such as binder concentration, shear force, and drying conditions, all of which impact the final granule size and moisture level.3 Blending is susceptible to segregation, where poor blend uniformity can result in tablet dose variability in downstream OSD processes.4 Coating processes depend on spray rate and uniformity, making the entire system vulnerable to fluctuations at any stage.
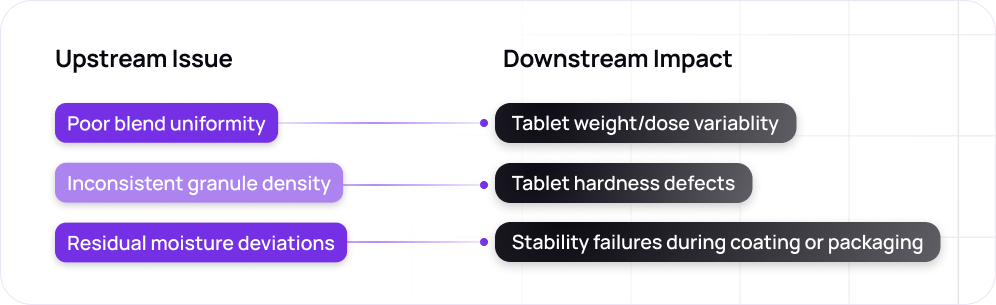
Continuous processing can further exacerbate these challenges, as maintaining "plug flow" (essential for uniformity) requires precise alignment of equipment with material characteristics.4
Conventional data-driven approaches, while useful for retrospective analysis, are often insufficient to address this level of variability in real time. These multivariate models (e.g. MVA) may capture the "golden batch" trajectory but do not offer recommendations or automatically adjust set points when deviations are detected. Further, these data-driven models are often “black boxes”, and do not offer any engineering “explainability” regarding the root cause of deviations or other quality issues.
In other words, with the existing approaches taken today, manufacturers are often alerted to issues, but lack clear guidance on how to resolve these problems in a way that regulators and engineers can understand and accept.
Where OSD Manufacturing Stands Today
Despite advances in automation and PAT (Process Analytical Technology), many pharmaceutical companies still operate with segmented data systems, legacy equipment, and reactive quality control protocols.
When a batch fails to meet specifications, root cause investigations often rely on manual data aggregation and trial-and-error adjustments. This reactive approach can result in prolonged downtimes, yield losses, and potential compliance risks.5
There is a growing recognition that modern OSD manufacturing demands a more proactive, predictive framework—one that can simulate outcomes before they happen, recommend the optimal path forward, and learn continuously from process data.
AI Applications for Reducing Variability in OSD Manufacturing
AI introduces a transformative approach by addressing the root causes of variability across the OSD manufacturing process. Rather than relying solely on descriptive analysis, true process digital twins can continuously learn from historical and real-time data, simulate outcomes, and recommend changes that align with quality and performance targets.
AI-Enabled Digital Twins for Process Simulation
A digital twin is a real-time virtual representation of a physical process, in OSD manufacturing it mimics the behavior of granulators, blenders, tablet presses, and coaters under a variety of conditions. These AI-enabled systems can allow manufacturers to achieve a variety of applications, such as simulating “what-if” scenarios without interrupting production. A hybrid digital twin model can take simulation further by combining physics principles with data-driven approaches for improved model accuracy.
For example, a digital twin of a blending process can simulate how varying impeller speed or blend time influences the uniformity of API distribution across a batch. Instead of relying on post-blend assay results to identify segregation or content uniformity issues, operators can use these predictive insights to proactively adjust parameters and ensure consistent blend quality before production begins.6
Studies have shown that model-based simulations can significantly enhance the understanding of powder mixing dynamics, reducing reliance on trial-and-error methods and improving batch consistency.7
Digital twins are particularly valuable for scale-up and tech transfer, allowing teams to test process adjustments across equipment and manufacturing sites while minimizing the risk of inconsistencies.
Real-Time Process Monitoring and Optimization
One of the most powerful uses of AI in OSD manufacturing is its ability to monitor, predict, and optimize processes in real time. Combining digital twins with AI optimizers allows for the ingestion of data from sensors (PAT tools, MES systems, etc.) to detect early signs of deviation and provide recommendations to adjust process setpoints before issues impact product quality.
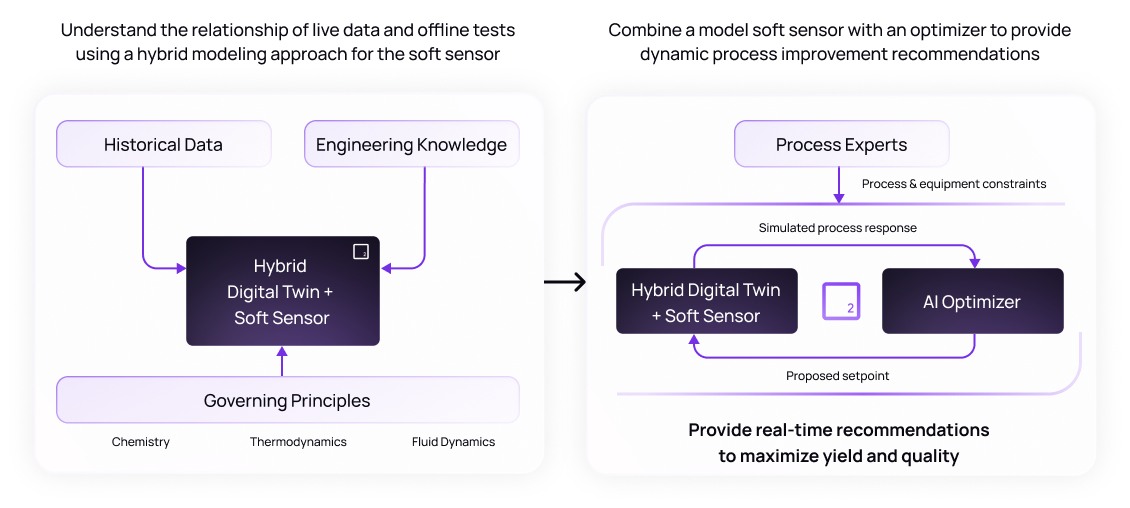
Unlike conventional control systems, AI doesn’t just flag anomalies, it recommends setpoint adjustments or process interventions tailored to current conditions. For instance, in one use case by Basetwo granulation moisture begins trending outside the desired range identified by the digital twin, the AI system then suggests modifications to the drying air temperature and the flow rate to correct the issue.8
This shift from reactive to prescriptive control enhances process reliability and ensures that each stage stays within critical quality attributes, even in the face of raw material variation or equipment wear.
Conclusion
Maintaining consistency in oral solid dose manufacturing is both a regulatory imperative and a business necessity. With quality and compliance on the line, pharmaceutical manufacturers can no longer afford to rely on legacy tools that offer limited foresight and delayed responses.
AI and digital twins offer a fundamentally different approach—one that combines predictive insights, real-time monitoring, and prescriptive control to reduce variability at its source.
By embracing modern technologies such as digital twins and AI, OSD manufacturers can not only meet today’s production demands but also position themselves for long-term success in an increasingly complex regulatory and competitive landscape.
To explore how AI can impact your OSD processes, book a demo with Basetwo today!
References:
- U.S. Food and Drug Administration. (2021). Guidance for Industry: Process Validation: General Principles and Practices. https://www.fda.gov/media/71021/download
- Zhao, Y., et al. (2020). Impact of API and excipient variability on blend uniformity in tablet manufacturing. International Journal of Pharmaceutics, 586, 119564.
- Singh, R., et al. (2019). Process understanding and control in pharmaceutical granulation: A review. Powder Technology, 356, 478-495.
- Davila, H. T. (2013, April 16). How to optimize continuous OSD manufacturing. Pharma Manufacturing. https://www.pharmamanufacturing.com/facilities/op-ex-lean-six-sigma/article/11357740/pharma-industry-how-to-optimize-continuous-osd-manufacturing-pharmaceutical-manufacturing
- Kumar, A., et al. (2021). Challenges in implementing real-time release testing and AI in pharmaceutical manufacturing. Journal of Pharmaceutical Innovation, 16, 155-167.
- Huang, Y.-S. (2023, July 25). Digital Twin Development and advanced process control for continuous pharmaceutical manufacturing. figshare. https://hammer.purdue.edu/articles/thesis/Digital_Twin_Development_and_Advanced_Process_Control_for_Continuous_Pharmaceutical_Manufacturing/23735379%C2%A0
- Garcia-Munoz, S., Bonam, R., & Goyal, S. (2020). Application of digital twins in pharmaceutical development and manufacturing. AAPS Journal, 22(5), 1–10.
- Pharmaceutical manufacturing: Basetwo Ai. Pharmaceutical Manufacturing | Basetwo AI. (n.d.). https://www.basetwo.ai/pharmaceuticals