In the aerospace industry, the assembly line is a critical component of the production process, to construct aircraft, spacecraft, and other flight systems efficiently and with high precision.
Given the complexity of the process, achieving assembly line balance is essential to optimizing productivity, reducing costs, and maintaining high-quality standards.
This article explores the importance of assembly line balancing in aircraft assembly, outlining the common challenges manufacturers face and examining AI-powered methods for solving the Assembly Line Balancing Problem (ALBP).
The Assembly Process for the Boeing 787 Dreamliner
To put the importance and complexity of line balancing into context, we can take a look at the manufacturing process for the Boeing 787 Dreamliner aircraft.
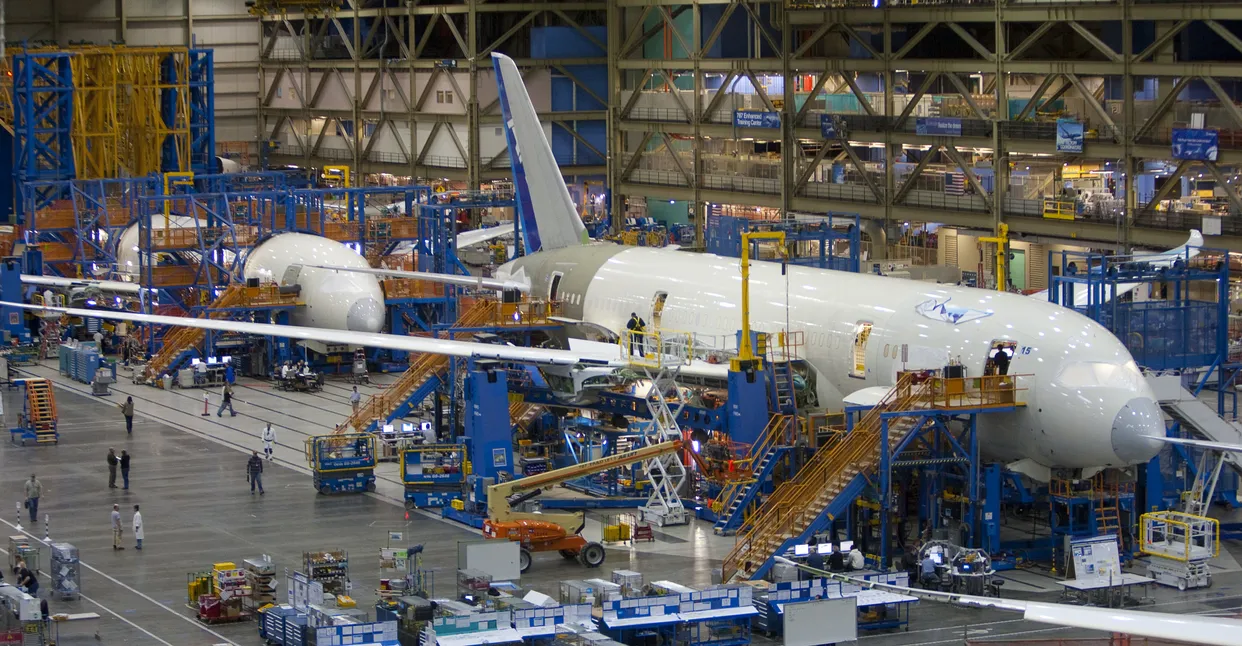
The 787 employs a distributed manufacturing approach, meaning major aircraft components are produced by subcontractors worldwide, including wings from Japan, fuselage sections from Italy and Japan, and doors from France and Sweden.1
After the components are shipped to the factory in North Charleston, South Carolina, several sub-assemblies are prepared. The midbody is assembled first, followed by the aftbody, which involves constructing the rear fuselage sections. Once complete, the interiors assembly focuses on installing key internal components like panels, storage bins, and cabin equipment.
Finally, the sub-assembled fuselage sections are brought together on the final assembly line, where up to eight 787s can be built simultaneously, completing the complex orchestration of the entire manufacturing process.2
The global nature of this supply chain coupled with the complexity of aircraft assembly showcases the importance of line balancing. A precise balancing of tasks and resources ensures a smooth and even distribution of workload that avoids bottlenecks, this ensures that the maximum amount of 787s can be produced while enhancing quality in the most cost-effective way.
Problems Manufacturers Face with Line Balancing
Ensuring Quality at Each Stage of the Assembly Line
Each stage of assembly undergoes rigorous inspection and quality checks; however, non-conformance events can be difficult to identify and may disrupt the entire assembly line, causing delays in subsequent tasks.3
An added difficulty in balancing quality and efficiency is that problems uncovered during inspections often require rework, which can cascade and affect numerous downstream tasks, further complicating the assembly process.3
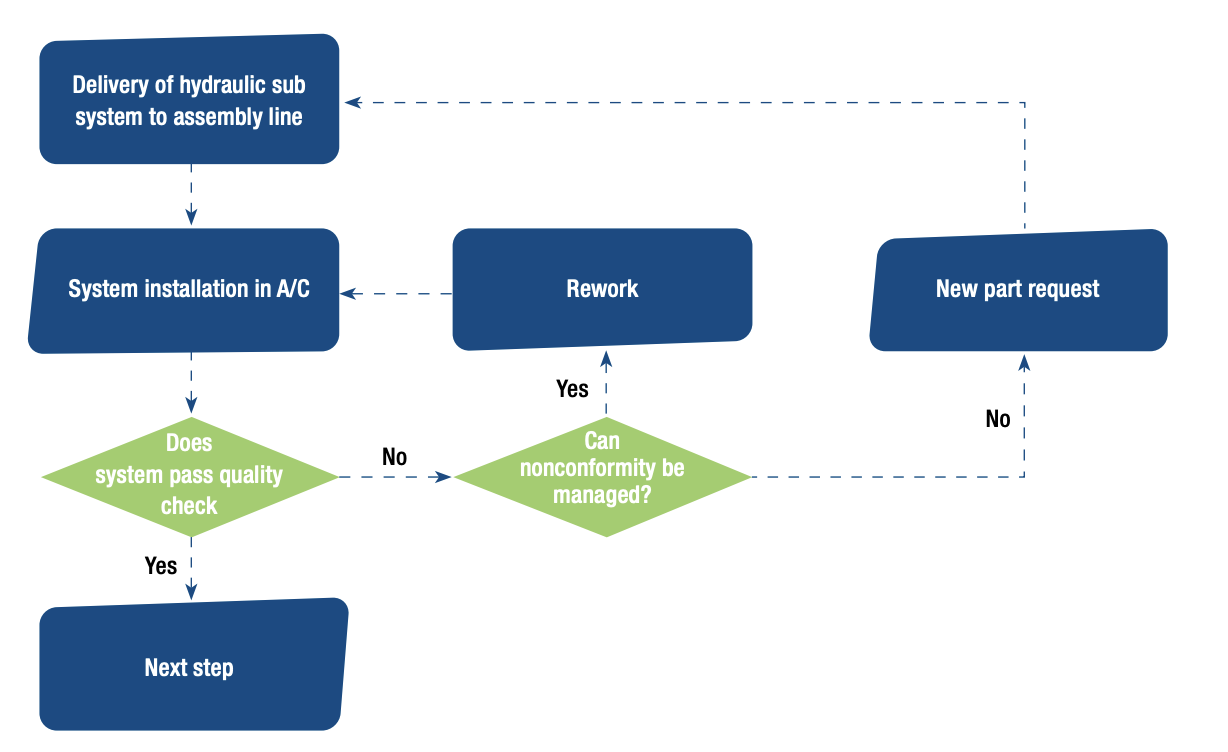
Real-time visibility into emerging issues and taking proactive steps to prevent non-conformance are essential for maintaining high quality and keeping the production line running smoothly without disruptions.
The Interdependent Nature of Tasks
Aircraft assembly is a highly sequential process where many tasks cannot begin until preceding tasks are completed, making it difficult to balance workloads across workstations while adhering to precedence constraints.4
For example, the Boeing 787 assembly line follows a critical path, ensuring that each component is installed in the proper sequence to prevent rework and delays. A delay in the assembly of major components like the fuselage, wings, or tail can have a ripple effect, pushing back subsequent tasks such as the installation of the electrical wiring.2
A variety of unforeseen challenges can disrupt this process, from tasks taking longer than anticipated to breakdowns in essential equipment. Even a minor delay in one task can quickly cascade, causing bottlenecks and creating widespread delays across the entire assembly line.
To address this, manufacturers must carefully plan their assembly line balancing to not only be efficient but also to avoid overburdening any one workstation. This allows for greater flexibility in the process and ensures that no workstation is running at full capacity, which could otherwise lead to bottlenecks, increased equipment wear, and reduced ability to handle unexpected disruptions.5

Resource Availability & Supply Chain Issues
In the production process for the 787, the timely arrival of components from Japan, Italy and France is crucial for maintaining the assembly schedule. Limited availability of resources, low inventory levels, or delays in the arrival of parts, create bottlenecks, delaying the entire assembly process.
Similarly, on the assembly line, the availability of skilled workers and specialized equipment must be available at precise times. Delays in one area can lead to idle resources in another, affecting overall efficiency. Manufacturers are challenged to balance these constraints when assigning tasks to workstations to avoid overloading certain areas while underutilizing others.6
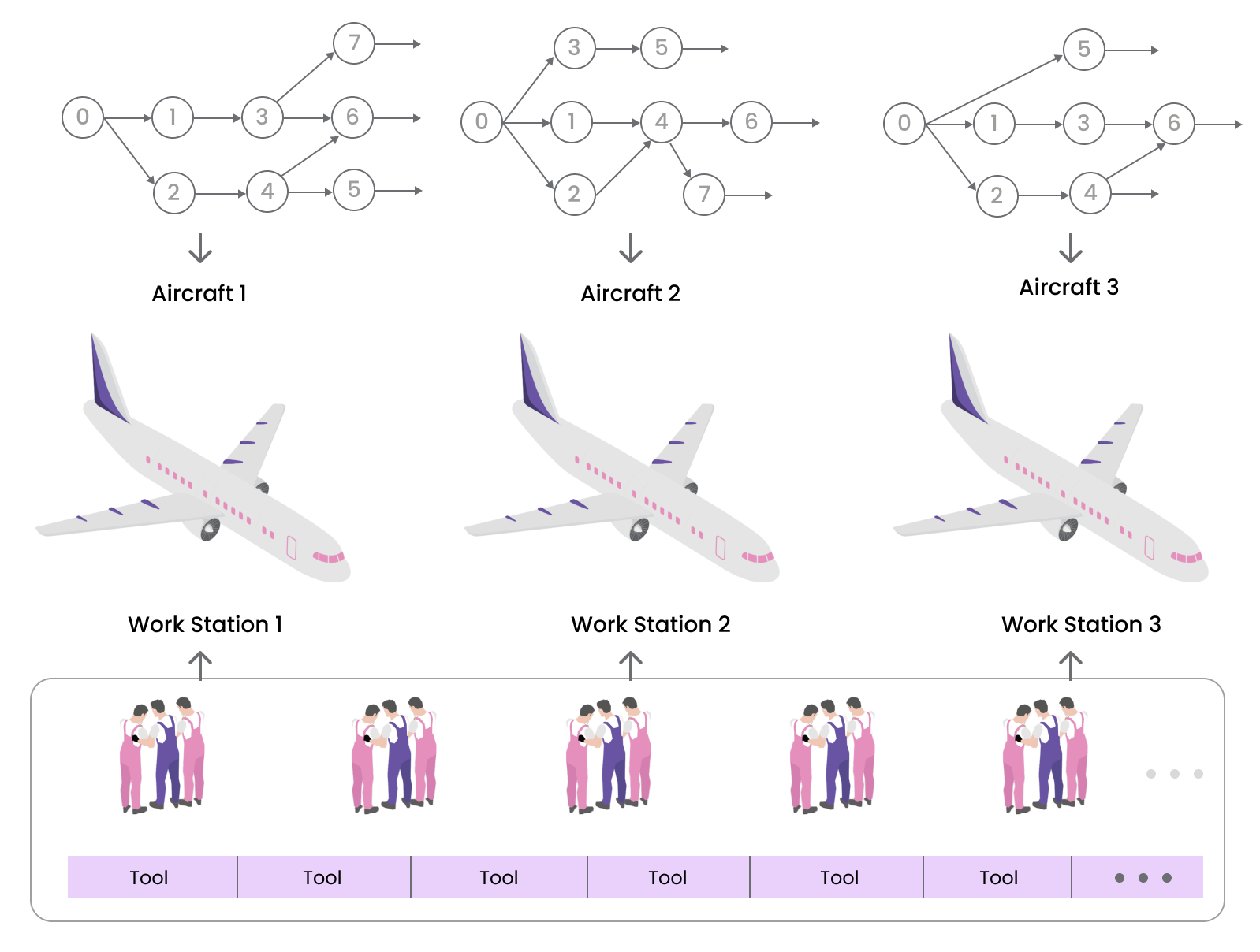
Driving Productivity with Keeping Costs Down
Assembly can make up as much as 50% of the total production timeline and over 20% of the overall manufacturing costs for aircraft. For this reason, efficiently balancing assembly lines is crucial to enhancing profitability and maintaining competitiveness for aircraft manufacturers.7
These costs can be attributed to equipment, labour, setup, idle time, and reconfiguration of the assembly line, with equipment and labour being the primary costs.8
Balancing the assembly line while minimizing costs across these areas is a constant challenge for manufacturers.
AI-Enabled Methods for an Optimized Line Balancing Process
Part 1: Simulation of Aircraft Assembly Lines
One effective approach to optimizing line balancing for ALBP is to simulate the process through the use of digital twins. By creating a virtual model of the assembly line, manufacturers can simulate different scenarios to identify potential bottlenecks and streamline workflows.
Due to the variability and uncertainty in aircraft assembly, simulations can support manufacturers in a proactive approach to line balancing. By modeling stochastic elements like variable task completion times, equipment breakdowns, material delays, and worker availability, manufacturers gain a more accurate assessment of line performance.10
Manufacturers can utilize discrete event simulation (DES)—a digital twin model that simulates specific events at distinct moments in time11 —to include precedence networks for mapping out task dependencies while considering the stochastic elements mentioned above.12
The primary advantage of simulation and virtual experimentation is that it allows manufacturers to explore how the manufacturing line behaves and how it responds to changes. It increases process understanding and also provides insights into efficient ways to balance the assembly line without needing to alter the physical plant.13
Part 2: Optimization of Assembly Lines
Optimization takes simulation a step further by leveraging the virtual replication of the assembly line process to explore the most efficient way to balance the line.
By leveraging multi-objective optimization, manufacturers can achieve multiple goals simultaneously through solving ALBP, such as minimizing the cycle time (and simultaneously increasing productivity rate), lowering equipment costs, and ensuring tasks are distributed smoothly across the assembly line.
AI-powered modeling enables manufacturers to achieve these objectives while accounting for key constraints like setup times between tasks, operation durations, and task dependencies.14 In one case study using DES to make small adjustments and improvements to a production line, productivity was increased by nearly 400%.15
As a real-world example, we can explore the paper “A Digital Twin-based approach to the real-time assembly line balancing problem” (Ragazzini, L. et al., 2021). In this paper, a digital twin was created with real-time data to simulate an operator being unavailable at a workstation for 45 minutes. Normally, this would cause the workstation’s utilization to spike close to its maximum capacity, which would delay the entire assembly. However, with real-time optimization, the impact was significantly reduced, keeping the workstation’s utilization at a manageable level, minimizing delays, and maintaining efficiency.12
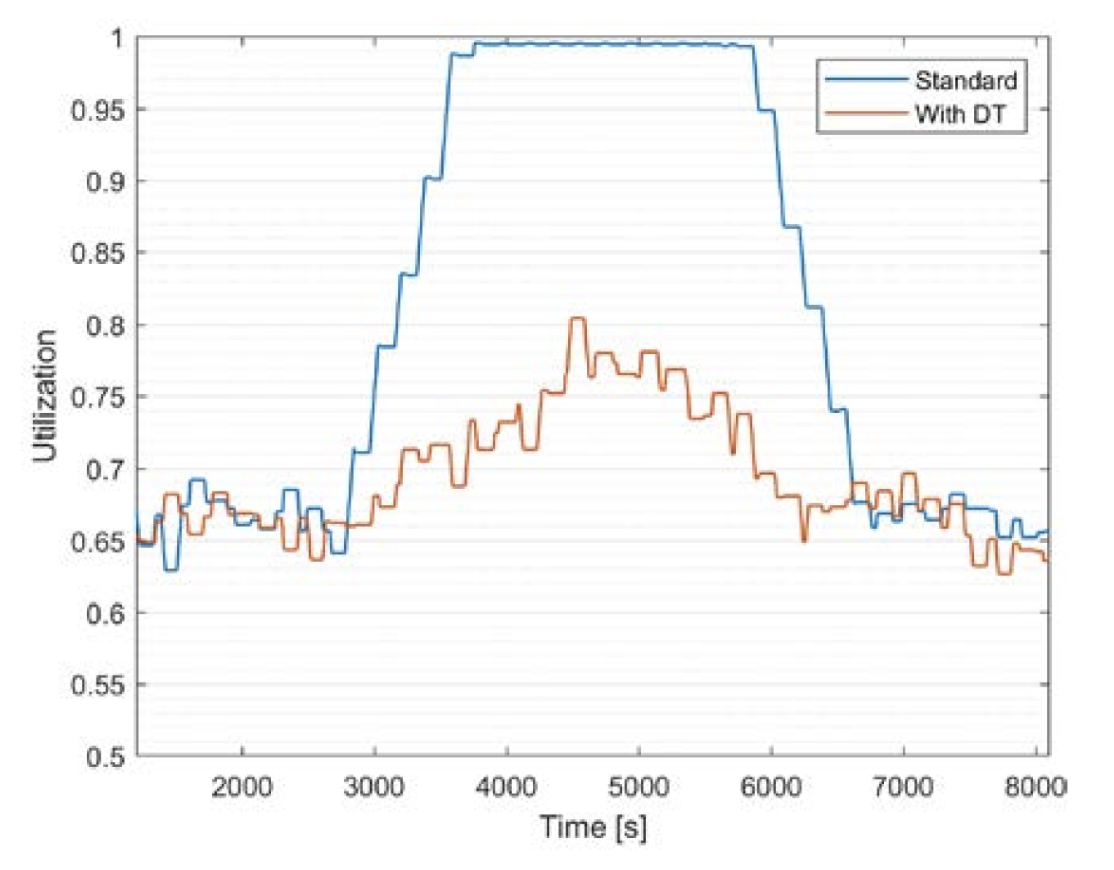
Tools like Basetwo enable manufacturers to run numerous virtual "what if" scenarios, helping them identify the optimal strategies for balancing the line while maximizing productivity and minimizing costs.
Conclusion
In the aerospace industry, where precision and efficiency are paramount, achieving proper assembly line balancing is critical to success. The complex and highly interdependent nature of aircraft manufacturing, as seen in the Boeing 787 Dreamliner’s assembly process, highlights the importance of maintaining a smooth flow of production. With numerous factors to consider—from resource availability to labor constraints, equipment costs, and quality control—manufacturers face constant challenges in balancing productivity, costs, and quality.
By leveraging these AI-driven approaches, aerospace manufacturers can enhance the overall performance of their assembly lines—improving productivity, controlling costs, and maintaining the high standards of quality required for aircraft production.
If you're looking to optimize your assembly line balancing, reach out to Basetwo and explore whats possible for your team.